Unraveling Causality in Neuroscience
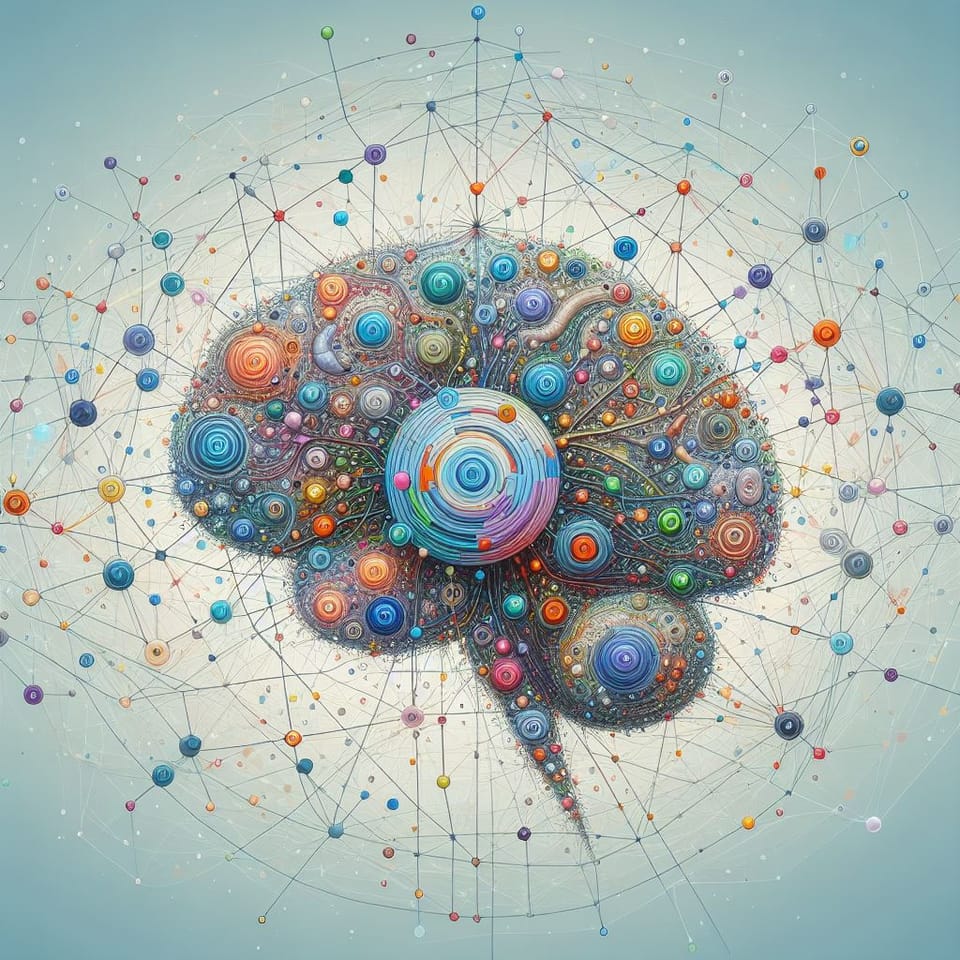
Hey Neurons, this week I've been contemplating causality in Neuroscience, realizing the complexity of making causal claims. Working with numerous neurons across various brain regions poses challenges when attributing influence from one region to another, often involving multiple interconnected areas. Consequently, creating models of the entire brain typically relies on correlation analysis. I'd like to share a couple of lectures I recently watched on this subject, hoping they'll deepen your understanding. Best of luck in your learning journey!
đź‘€ Something to watch
đź‘€ Lecture series - Causality in Minds and Machines
One of my favorite lecturers, Prof. Lauren Ross, presented in this lecture series on causality in minds and machines. Causality plays an important role in science, as it allows us to infer which variables influence one another. In general, there is often confusion between correlation and causation. The lectures highlight how people, including scientists, often mistake correlation (when two variables co-occur) for causation (when one variable directly influences the other). In the first talk, Prof. Ross explains the utility of the concept of causation in science:
- Explanation: Causation is pivotal for explaining phenomena; it underlies most scientific explanations, as causes explain their effects.
- Prediction: Identifying causes enables predictions of future events based on the known causes.
- Control: Causes provide control over their effects, essential for altering outcomes, be it in scientific contexts or everyday life.
- Attributing Responsibility: Causation guides attributions of responsibility, essential in various scenarios like determining the causes of a car crash.
I highly enjoyed listening to this lecture series and would recommend it to any student interested in creating neuroscientific models for understanding the brain.
🎧 Something to listen
🎧 Podcast - Judea Pearl on Cause and Effect
A few years back, I took a course where I delved into Judea Pearl's book called 'the book of why', and it completely shifted my perspective on causal modeling. In the realm of imaging neuroscience, our primary focus tends to revolve around correlation, because altering the system without adversely affecting the participants is challenging. Nevertheless, there's a path toward crafting causal models through targeted research utilizing animal models like mice. In these models, we can directly impact brain activity using methods such as deep brain stimulation (DBS). In the future, this type of research can aid in optimizing DBS protocols for human participants.
📚 Something to read
📚 Paper - The Brain is…”: A Survey of The Brain’s Many Definitions
This article delves into the perspectives through which scientists define the brain within academic literature. Employing text analysis tools, it records expressions starting with “the brain is…” present in scientific writings. I found the exploration of the diverse definitions of the brain highly interesting. Moreover, this diversity underscores the challenge of consolidating distinct viewpoints about the brain—whether it is called a prediction machine or a vascularized organ. Undoubtedly, the brain embodies elements of both analogies, yet emphasizing one tends to overshadow other aspects of this complex organ. For instance, when we call the brain a computer, it overlooks the brain's nature as an organ with its unique biochemical properties. Thus, even though the brain potentially shares certain functionalities with a computer, it fundamentally differs due to its biological essence.
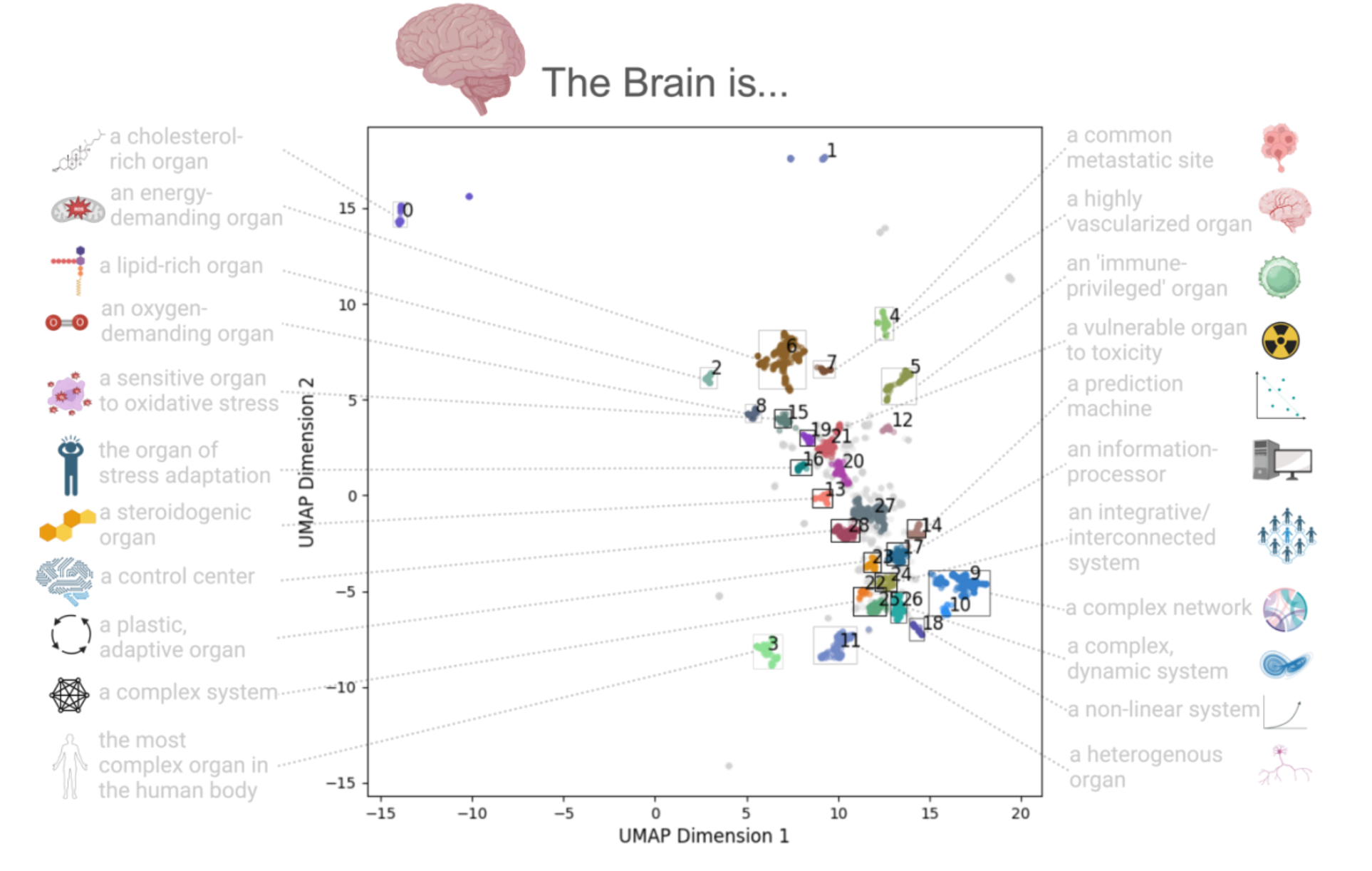
👩‍💻 Weekly Quote
“That's just what translation is, I think. That's all speaking is. Listening to the other and trying to see past your own biases to glimpse what they're trying to say. Showing yourself to the world, and hoping someone else understands.”
― R.F. Kuang, Babel