Machine Learning in Psychiatry: Bridging the Gap between Data and Diagnostics
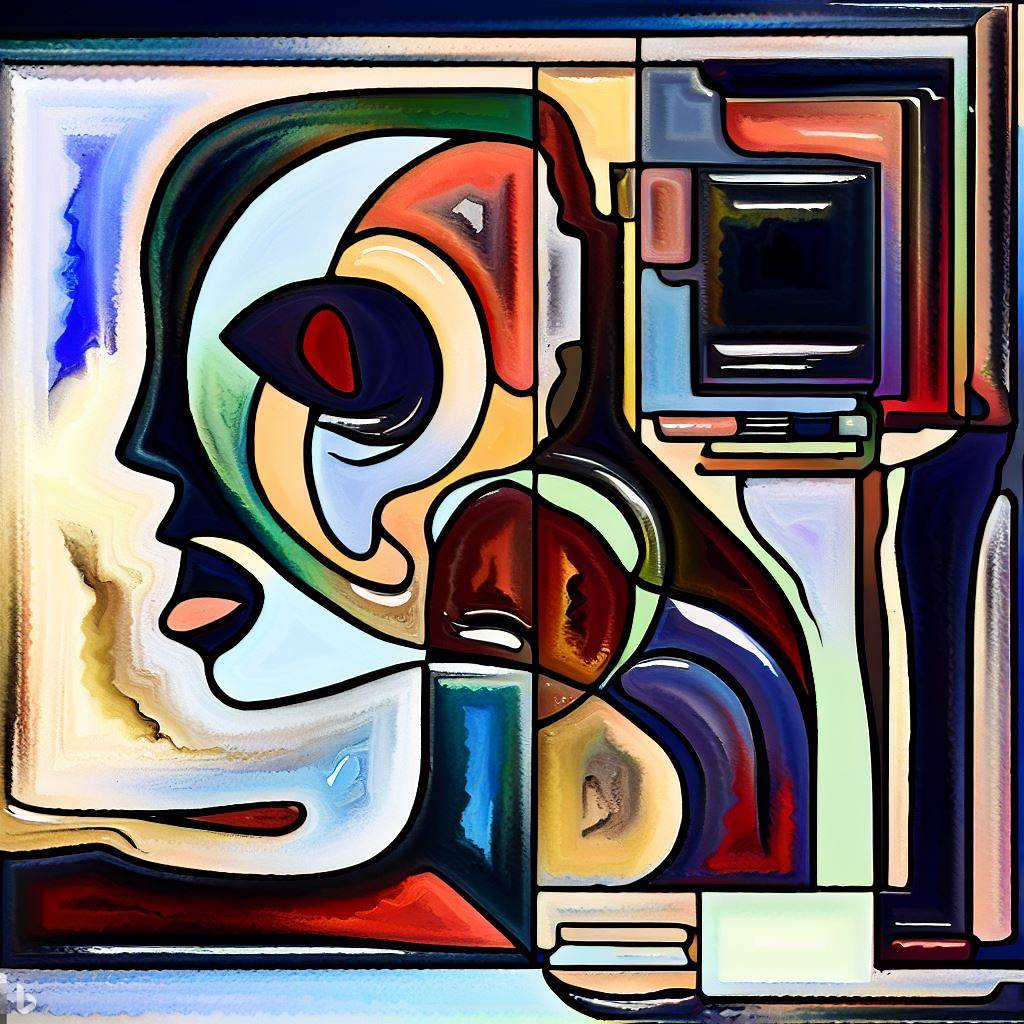
Hey Neurons,
As this week unfolds, I find myself engrossed in the captivating world of computational psychiatry in Zurich. Within the realm of this course, I'm diving into diverse machine-learning techniques employed within psychiatry. These techniques shed light on the intricate mechanisms underlying various mental disorders. Today, I'm excited to extend a glimpse into the concepts I explained during my lecture, offering you resources to delve deeper into this fascinating subject if you're inclined to do so.
In the broader context of applying machine learning to neuroscience, we can distill the intricate process into three pivotal stages: Feature extraction/selection, model fitting through classification/regression via cross-validation, and the crucial task of evaluating performance.
While each of these stages is substantial enough to warrant its own dedicated lecture series, let me provide you with a concise overview:
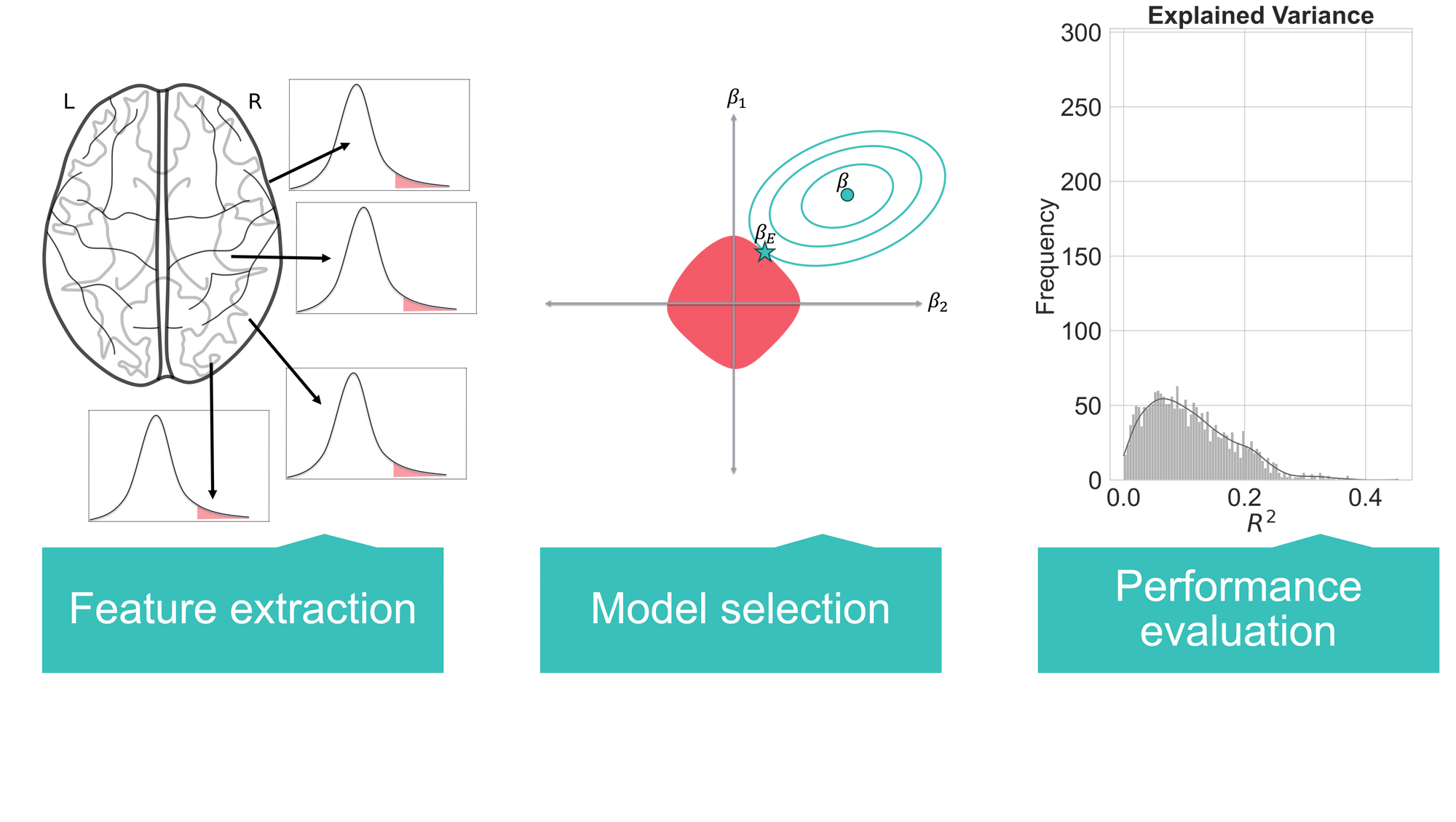
Feature Extraction and Selection: Here, the first decision revolves around whether to construct an all-encompassing model of the brain, provided you possess the computational firepower to tackle such complexity. However, it's crucial to be wary of the potential for overfitting due to the high dimensionality of the feature space. Another approach involves targeting specific regions of interestāareas hypothesized to encode the most compelling signals in your data. For instance, in the study of memory, the hippocampus might take the spotlight. Alternatively, you could fashion your own features via manifold learning techniques, such as principal component analysis (PCA) or independent component analysis (ICA).
Selecting Classification/Regression Algorithms: When confronted with a classification challenge, such as distinguishing between patients and controls based on brain features, the choice of algorithm takes center stage. An array of approaches awaits, including regularization methods, probabilistic strategies, ensemble techniques, and the ever-potent neural networks. It's vital to comprehend that no single approach is the best. The most suitable choice hinges on your specific inquiry and the nature of your data. Furthermore, it's essential to remain mindful of the assumptions inherent in each model.
Statistical Assessment of Model Performance: Finally, as you wrap up your analysis, the crucial task of reporting error metrics awaits. To make informed decisions, keep in mind that different metrics are sensitive to distinct facets of your data. For instance, the Mean Squared Error (MSE) is contingent on the data's scale. Therefore, presenting a diverse array of error statistics that comprehensively encapsulate various facets of your model's fitness holds paramount importance.
šSomething to read
If you are excited to learn more about computational psychiatry and its possible application. I highly recommend this reading list from the CPC Zurich course. It goes from brain disease models of addiction to the free-energy framework for perception. Slowly working through this reading list will give you immense insights into what is possible currently and where questions remain. Ā
š§ Happy coding neurons!